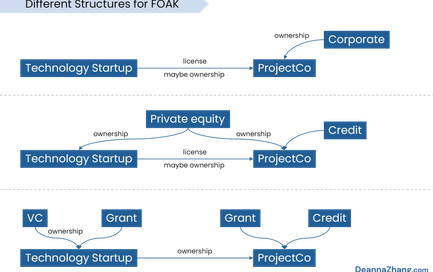
First post in a while! Thank you all for being patient with me.
Today I want to share some thoughts on data in FOAK projects. Christian Okoye, Caleb Cunningham, and I have been working through a series of posts on the subject. See below for the first two:
PART 1 - Why data is important in FOAK
PART 2 - What data is important in FOAK
Why I care about this:
My day-to-day is working alongside startups preparing to raise money for their FOAK projects. One of the first major issues I see in almost all of the startups that I come across is the lack of an adequate financial model. Putting aside common problems like readability, not having project economics, a confusing model structure, etc., most startups just don't sensitize their numbers enough (or worse, at all). They don't run scenarios on what happens if the offtake pricing comes in at a 15% discount or if the construction costs come in 30% above their FEL3 estimate or if their equipment delivery time stretches by 6 months longer than anticipated. They don't build out the scenario that payment from their product gets taken on delivery vs. on production or that cash from the tax credits comes in much later than anticipated. They don't build in the case of an unanticipated equipment failure or longer-than-expected turnaround time.
This is a HUGE issue for FOAK projects because things will go wrong & not running the right scenario analysis often means not being prepared for when things do go wrong. The reason why most startups don't do this is not due to inherent inadequacy (we often point to founders being unfailingly optimistic as a character flaw), it's due to the lack of knowledge of what CAN go wrong & the boundary conditions for those downside scenarios.
This is where data can be powerful. Data on past projects can tell us both: what scenarios should we be running and what boundary conditions should we be running in those scenarios.
It will make my job a lot easier too. Part of my job coming into a startup preparing for a FOAK fundraise is to ask the hard questions that define the risks around a project & see what we can do to mitigate those risks before the fundraise. With data, we can better define what's likely or not with actual statistics. And I can make a stronger argument for why it's important to ask these hard questions.
Look forward to continuing to work on this issue & thanks again to Christian & Caleb for starting this crazy cool initiative. If anyone has some of this data and would like to work on this mission with us, please reach out.